DataRobot is transforming the way businesses approach artificial intelligence and machine learning. As one of the leading platforms for automating AI processes, DataRobot simplifies the entire machine learning lifecycle—from data preparation to model deployment. Its powerful AutoML capabilities enable data scientists and non-experts to quickly build, train, and deploy models with minimal effort, unlocking valuable insights and driving smarter decision-making. In this article, we will explore how DataRobot is revolutionizing the field of AI. These key features set it apart, and why it has become a go-to solution for enterprises across various industries.
What is DataRobot?
DataRobot is an advanced platform designed to automate the machine learning process from start to finish. It allows users to build, train, and deploy machine learning models quickly and efficiently, even without deep expertise in data science. By leveraging AutoML, DataRobot simplifies tasks like data preparation, model selection, and hyperparameter tuning, saving time and reducing the complexity of traditional machine learning workflows.
With its wide range of algorithms and support for both structured and unstructured data, DataRobot is suitable for various use cases across industries like finance, healthcare, and retail. One of the platform’s key strengths is its ability to handle large datasets and complex models, ensuring that businesses can scale their AI initiatives effectively. Additionally, DataRobot focuses on providing explainability and transparency, helping users understand how models make predictions, which is crucial for building trust in AI systems.
By integrating seamlessly with popular cloud platforms and enterprise tools, DataRobot has become a powerful solution for organizations looking to harness the full potential of AI. It also offers robust MLOps capabilities, ensuring that models remain accurate and up-to-date over time, even in dynamic environments. DataRobot is a comprehensive tool that empowers businesses to accelerate their AI-driven innovation.
An Overview of DataRobot and its Role in AI Automation
DataRobot is an advanced platform that automates the end-to-end machine learning process, making AI more accessible to businesses of all sizes. By offering a wide range of built-in algorithms and tools for data preparation, model building, and deployment, DataRobot significantly reduces the time and expertise needed to create effective machine-learning models. One of the platform’s key advantages is its AutoML capabilities, which automatically select the best algorithms, optimize hyperparameters, and generate insights, allowing even non-experts to build high-performing models.
DataRobot plays a crucial role in AI automation by streamlining tasks that traditionally require data scientists and specialized skills. From predictive analytics to time series forecasting, the platform enables businesses to leverage AI in real-time decision-making, ultimately increasing efficiency and scalability. Moreover, its robust MLOps features ensure continuous monitoring, retraining, and governance of deployed models, making AI projects sustainable over time.
By simplifying complex machine learning tasks, DataRobot helps organizations focus on using insights to drive business growth rather than getting caught up in technical hurdles.
How DataRobot is Revolutionizing Machine Learning
DataRobot is revolutionizing machine learning by automating the most complex and time-consuming parts of the process. Traditionally, building machine learning models required deep technical expertise and hours of manual effort to prepare data, select algorithms, tune hyperparameters, and evaluate results. DataRobot changes this by leveraging AutoML (Automated Machine Learning) technology, allowing users to create models quickly and accurately without needing advanced coding skills.
With DataRobot, businesses can automatically test dozens of algorithms and frameworks, ensuring the best model is selected for their specific data. It also simplifies hyperparameter tuning, a process that typically requires a lot of trial and error. This automation significantly reduces the time to deploy AI solutions, enabling faster insights and decision-making.
Additionally, DataRobot offers built-in explainability tools, making it easier for users to understand and trust their models’ predictions. This transparency is crucial in industries like finance and healthcare, where trust in AI-driven decisions is vital.
DataRobot is not just about speed and automation—it’s also about scalability. It can handle vast datasets, support multiple deployment options (cloud or on-premise), and monitor models in real time to ensure they perform optimally over time.
In short, DataRobot is making machine learning more accessible, efficient, and scalable, empowering companies to harness the power of AI without the usual technical barriers.
Key Features of DataRobot
DataRobot offers a powerful set of features that make it a standout platform for automating machine learning and AI processes. These features help simplify complex tasks, enabling users to build, train, and deploy models efficiently. Whether you’re a seasoned data scientist or just starting, DataRobot provides the tools you need to leverage AI effectively.
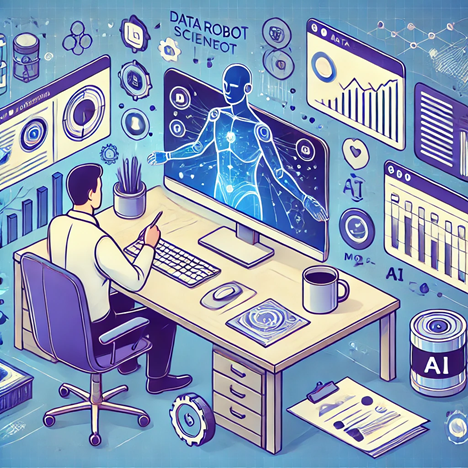
Here are some of the key features of DataRobot:
- AutoML
DataRobot automates the entire machine learning process, from data preparation to model selection, training, and evaluation. This significantly reduces the time and effort required to develop high-quality models. - Wide Range of Algorithms
The platform supports a vast library of algorithms, including decision trees, random forests, deep learning models, and time series forecasting. DataRobot automatically chooses the best algorithm for your dataset, saving you from manually testing different options. - Explainable AI
One of the standout features is the platform’s focus on explainability. With built-in tools for model interpretation, DataRobot helps users understand how their models make predictions, ensuring transparency and trust in AI-driven decisions. - Model Deployment
Deploying models with DataRobot is seamless. You can deploy models into production with just a few clicks, and the platform also supports both real-time and batch prediction services. - MLOps
DataRobot includes comprehensive MLOps features, which allow for continuous monitoring, model management, and retraining. This ensures that deployed models stay up-to-date and perform optimally over time. - Integration with Other Tools
The platform easily integrates with popular data sources, cloud platforms, and business tools like Salesforce, Snowflake, and Tableau, ensuring smooth workflows and making it easier to incorporate machine learning into existing systems.
These features, combined with DataRobot’s user-friendly interface and robust capabilities, make it an excellent choice for businesses looking to accelerate their AI initiatives.
AutoML: Simplifying Machine Learning Workflows
AutoML, or Automated Machine Learning, is revolutionizing how organizations approach machine learning by automating many of the complex and time-consuming tasks traditionally handled by data scientists. With AutoML, platforms like DataRobot enable users to automatically select the best machine learning models, optimize hyperparameters, and handle tasks like data cleaning and feature engineering. This automation significantly reduces the time and expertise needed to build effective machine-learning models.
One of the key benefits of AutoML is that it allows non-experts to leverage advanced machine-learning techniques without needing to master coding or complex algorithms. DataRobot, for example, streamlines the entire process, allowing users to focus on business insights rather than the technical details of model creation.
By simplifying these workflows, AutoML democratizes AI, making it accessible to a broader range of professionals and accelerating the adoption of machine learning across industries. It ensures faster development, more accurate models, and easier deployment, all while reducing the risk of human error.
For those looking to incorporate machine learning into their organization, AutoML is an invaluable tool that makes the process more efficient and scalable.
Comprehensive Algorithm Library: From Regression to Deep Learning
DataRobot offers a comprehensive algorithm library that spans a wide range of machine learning techniques, from simple regression models to complex deep learning architectures. This allows users to apply the right algorithm to their specific data and use case, ensuring optimal results.
For traditional machine learning tasks, regression models are available for predicting numerical values, while classification algorithms can handle tasks like sorting data into categories. For more advanced needs, DataRobot provides algorithms like Random Forest, Gradient Boosting Machines (GBM), and XGBoost, which are great for handling large datasets with many variables.
On the deep learning side, DataRobot supports neural networks, which are ideal for tasks such as image recognition, natural language processing, and time series forecasting. The platform’s automated system selects, tests, and ranks these algorithms, so users can quickly identify the best-performing model for their data.
Whether you’re working on a simple task or a highly complex AI project, DataRobot’s algorithm library ensures that you have access to the most effective tools to get the job done.
Feature Engineering and Data Preparation Tools
Feature Engineering and Data Preparation Tools are crucial aspects of building accurate and effective machine learning models. DataRobot simplifies these processes by automating much of the feature engineering and data preparation work, allowing users to focus on refining their models rather than spending time on manual data processing.
Feature engineering involves transforming raw data into meaningful features that improve the model’s performance. This can include tasks like creating new variables, normalizing data, handling missing values, and encoding categorical data. DataRobot offers built-in tools to automate these tasks, ensuring that even users with limited experience in data science can efficiently prepare their datasets.
In addition to feature engineering, data preparation is a critical step. This involves cleaning and organizing the data to ensure it’s in the right format for model training. DataRobot integrates seamlessly with various data sources and offers preprocessing steps such as data normalization, outlier detection, and missing data imputation. By automating these processes, users can quickly prepare their data for analysis, reducing the time to insight.
In summary, these tools not only save time but also ensure higher model accuracy, enabling businesses to make better decisions based on well-prepared data.
Explainable AI: Ensuring Transparency and Trust in Models
Explainable AI is crucial for building trust in machine learning models, especially when these models are used in industries like healthcare, finance, and legal services, where decisions have a significant impact. Explainable AI ensures that users can understand how a model reaches its conclusions, making the decision-making process more transparent and accountable.
In DataRobot, explainable AI is integrated into the platform, providing tools that break down complex models into understandable insights. Users can see which features are most important to a model’s predictions, track how changes in input data affect outcomes, and detect any potential biases in the model. This transparency helps stakeholders feel confident in using AI-driven insights for critical decisions.
By making AI more interpretable, DataRobot not only increases trust but also allows organizations to meet regulatory requirements, such as those in finance, which often mandate explainability in algorithmic decision-making.
Having explainable AI builds a bridge between complex machine learning processes and practical, real-world applications, fostering wider adoption and confidence in AI solutions.
DataRobot’s Machine Learning Lifecycle
DataRobot’s Machine Learning Lifecycle refers to the entire process of developing machine learning models, from data preparation to deployment and monitoring. This lifecycle is designed to automate many of the tasks typically handled manually, making it faster and more efficient for businesses to implement AI solutions.
The process begins with data preparation, where raw data is cleaned, organized, and transformed into a format suitable for analysis. DataRobot provides tools to handle missing values, outliers, and categorical variables, ensuring the data is ready for modeling.
Next is automated model training. DataRobot evaluates multiple algorithms simultaneously to find the best-performing models for the dataset. This eliminates the need to manually test different approaches, saving time and effort.
After model training, the platform automatically handles hyperparameter optimization. This process involves fine-tuning the model’s settings to improve performance, all without requiring user intervention.
Once the model is optimized, it goes through the evaluation and validation phase. This step ensures the model generalizes well to new data and is ready for deployment.
Finally, DataRobot simplifies model deployment by offering one-click deployment options for both batch and real-time predictions. After deployment, models are continuously monitored to ensure they maintain accuracy and relevance.
This seamless automation of the machine learning lifecycle makes DataRobot an ideal choice for businesses looking to scale their AI efforts efficiently.
Data Preparation and Cleaning
Data preparation and cleaning are critical steps in the machine learning process, ensuring that the data used for model training is accurate, complete, and properly formatted. In DataRobot, this phase is largely automated, making it easier for users to handle messy or incomplete datasets. The platform identifies missing values, incorrect formats, and duplicates, and then offers built-in tools to clean and transform the data.
DataRobot also provides insights into potential issues, such as skewed distributions or outliers, allowing users to make necessary adjustments before moving forward with model training. Additionally, users can easily perform operations like normalization, encoding categorical variables, and scaling numerical features.
By automating much of the data cleaning process, DataRobot helps users save time and reduce the risk of errors, enabling them to focus on building accurate and effective models.
Automated Model Training and Selection
Automated Model Training and Selection is one of the key features that makes machine learning easier and faster. In traditional workflows, selecting the right algorithm and tuning it for optimal performance can be time-consuming and requires deep expertise. With automated model training, the process is streamlined.
DataRobot automatically trains multiple models on your data using a variety of algorithms, from linear regression to advanced deep learning models. It evaluates each model’s performance based on predefined metrics, ensuring the most suitable model is chosen for your specific task. This selection process is driven by AutoML, which helps users avoid the complexities of manual tuning while maintaining high accuracy and efficiency.
This automation not only saves time but also opens up the world of machine learning to users with less technical expertise, empowering more businesses to benefit from AI.
Hyperparameter Optimization and Tuning
Hyperparameter Optimization and Tuning is a critical step in improving the performance of machine learning models. Hyperparameters are the settings or configurations that control how a model learns from data, such as the learning rate or the number of layers in a neural network. Unlike model parameters, which are learned during training, hyperparameters must be set before the training process begins.
In this phase, different combinations of hyperparameters are tested to find the optimal settings that yield the best model accuracy. Effective tuning can significantly enhance the model’s predictive power and reduce overfitting or underfitting.
DataRobot simplifies hyperparameter tuning by automating the process. Instead of manually testing different settings, DataRobot runs multiple experiments in parallel, exploring various combinations of hyperparameters to identify the best configuration. This automated approach saves time and ensures that even complex models are optimized for performance.
Tuning is essential for achieving better accuracy and reliability in machine learning models, making it a key step in the overall model-building process.
Model Evaluation and Validation
Model Evaluation and Validation are crucial steps in the machine learning process to ensure that the model performs well and can generalize to new, unseen data. After training, the model is tested using a separate dataset to assess its accuracy and effectiveness. This is where metrics like accuracy, precision, recall, and F1-score come into play, helping to measure the model’s performance on different tasks.
In this phase, techniques such as cross-validation are often used to evaluate the model’s robustness. Cross-validation divides the data into subsets to test the model multiple times, providing a more accurate understanding of how the model will perform in real-world scenarios. By using these methods, potential issues like overfitting—where the model performs well on training data but poorly on new data—can be detected early.
DataRobot simplifies this process by automating the evaluation and validation steps, providing clear metrics and insights to help you understand how well your model is performing. You can trust these insights to fine-tune the model or decide whether to deploy it into production.
In machine learning, cross-validation is a powerful method to ensure reliable performance across different data samples.
Scalability and Performance
Scalability and performance are two key strengths that make DataRobot a preferred choice for many organizations. The platform is designed to handle a wide range of data sizes, from small datasets to massive, enterprise-level data, without compromising on speed or accuracy. Whether you’re running a simple regression model or a complex deep learning architecture, DataRobot efficiently allocates resources to ensure optimal performance.
In cloud environments, it leverages distributed computing to process large datasets quickly, enabling parallel processing across multiple machines. This means faster training times and the ability to scale as your data or computational needs grow. On-premise users can also benefit from this scalability by integrating DataRobot with their existing infrastructure, ensuring that performance remains consistent, even with high workloads.
By utilizing elastic resources, DataRobot adjusts to the needs of each task, ensuring that both small-scale projects and large-scale operations run smoothly. This makes it an ideal solution for businesses looking to scale their AI initiatives without worrying about infrastructure limitations.
Handling Large Datasets and Complex Models
Handling large datasets and complex models can be challenging, but DataRobot is designed to manage these tasks efficiently. Its platform supports distributed computing, allowing models to be trained on multiple machines simultaneously, which speeds up the process even when dealing with massive datasets. This scalability ensures that as data grows, the system can handle it without significant slowdowns.
When working with complex models, DataRobot automatically selects the best algorithms and tunes hyperparameters to optimize performance. It can also deploy deep learning models on GPUs for faster computation, especially for tasks like image recognition or natural language processing.
Additionally, DataRobot offers advanced parallel processing capabilities, allowing several models to be trained and evaluated at the same time. This not only saves time but also ensures that the best-performing model is selected for deployment. By combining distributed computing and parallel processing, DataRobot makes it possible to handle complex models and large datasets efficiently and effectively.
Distributed Computing and Parallel Processing
Distributed Computing and Parallel Processing are key features that allow machine learning platforms like DataRobot to handle large datasets and complex models efficiently. Instead of relying on a single machine to perform all computations, distributed computing splits the workload across multiple machines or nodes. This helps process massive amounts of data faster, as each node handles a portion of the task simultaneously.
Parallel processing takes this a step further by running multiple tasks at the same time within each node. For example, DataRobot can train several machine learning models in parallel, drastically reducing the time needed to test and evaluate different algorithms.
These technologies are crucial for industries dealing with big data, where traditional computing methods would be too slow or inefficient. By distributing tasks and running them in parallel, businesses can achieve faster insights and improve decision-making processes. Distributed computing is especially beneficial for large-scale applications, such as in finance or healthcare, where rapid data processing is critical.
Performance Across Cloud and On-Premise Deployments
Performance Across Cloud and On-Premise Deployments refers to the flexibility and efficiency with which DataRobot operates in both cloud-based and on-premise environments. In the cloud, DataRobot leverages the scalability and elasticity of platforms like AWS, Azure, and Google Cloud. This allows for handling large datasets, running multiple models in parallel, and scaling resources as needed, all without the limitations of physical hardware.
On-premise, DataRobot provides robust performance for organizations with specific data residency or security requirements. It can be deployed on local servers and integrates seamlessly with existing infrastructure, offering the same automation and AI capabilities without relying on cloud resources.
Whether in the cloud or on-premise, DataRobot ensures high-speed processing, efficient resource management, and optimal performance for both small and enterprise-scale machine learning projects.
Elasticity in the cloud allows organizations to adjust resources dynamically based on workload demand, enhancing cost-effectiveness.
Integration with Popular Platforms
Integration with Popular Platforms is one of the standout features of DataRobot, allowing seamless connectivity with various tools and services used by businesses. It easily integrates with major cloud platforms like AWS, Google Cloud, and Microsoft Azure, enabling users to access and analyze data stored in these environments. This integration allows for quick deployment of machine learning models directly into cloud infrastructures, ensuring scalability and performance.
Additionally, DataRobot connects with business platforms such as Salesforce, Snowflake, and Tableau, making it simple to incorporate AI insights into everyday workflows. For example, a company can use DataRobot to create predictive models and then visualize the results in Tableau, or automate decision-making processes in Salesforce. This flexibility ensures that organizations can leverage AI without disrupting their existing operations, enhancing productivity and efficiency.
By supporting a wide range of platforms, DataRobot ensures that businesses can unlock the full potential of AI across various applications.
Integrating DataRobot with AWS, Google Cloud, and Azure
Integrating DataRobot with cloud platforms like AWS, Google Cloud, and Azure provides businesses with the flexibility and scalability needed to handle large datasets and complex machine-learning workflows. These cloud platforms offer powerful infrastructure for storing and processing data, which complements DataRobot’s automated machine-learning capabilities.
By integrating with AWS, users can easily connect DataRobot to services like Amazon S3 for data storage or EC2 instances for scalable computing. Similarly, Google Cloud offers seamless access to BigQuery for data analytics and Google Cloud Storage, allowing for efficient data management and model deployment. Azure users benefit from integration with Azure Blob Storage and Azure Machine Learning, enabling smooth data transfers and large-scale model training.
These integrations help automate workflows, reduce the time spent on manual data handling, and improve performance when deploying models in the cloud. Each cloud platform’s native services work hand in hand with DataRobot to deliver a robust, scalable machine learning pipeline.
Using DataRobot with Salesforce, Snowflake, and Tableau
Using DataRobot with Salesforce, Snowflake, and Tableau enables businesses to integrate powerful machine-learning insights into their existing workflows seamlessly.
When integrated with Salesforce, DataRobot can enhance customer relationship management by providing predictive insights that help identify leads, forecast sales, and improve customer retention strategies. This allows teams to make data-driven decisions directly within the Salesforce platform.
With Snowflake, DataRobot connects to large-scale data storage systems, enabling efficient and scalable model training on massive datasets. Snowflake’s cloud data warehousing capabilities complement DataRobot’s AI tools, providing real-time access to high-quality data that can be used to train and optimize machine learning models.
Finally, integrating with Tableau allows DataRobot to visualize machine learning predictions and insights in an interactive and intuitive format. By embedding AI-driven analytics directly into Tableau dashboards, users can easily interpret model outcomes, uncover trends, and share findings with stakeholders for informed decision-making.
These integrations make it easier for organizations to operationalize AI, unlocking the full potential of their data while maintaining a familiar workflow.
Seamless API Integration for Custom Workflows
Seamless API Integration for Custom Workflows refers to how easily DataRobot can be integrated into existing systems and applications through its flexible API. By leveraging the powerful REST API, users can incorporate machine learning models into custom workflows, automating tasks such as data ingestion, model training, and predictions. This allows businesses to connect DataRobot with their software or third-party tools, ensuring smooth and efficient operations without needing manual intervention.
With the API, you can schedule automated model retraining, deploy models directly into production, and retrieve real-time predictions. It also supports the customization of workflows, enabling developers to build tailored solutions for specific business needs. For example, integrating DataRobot with CRM systems or data pipelines becomes straightforward, significantly reducing the time and effort required to maintain complex AI operations.
In short, DataRobot’s API integration empowers organizations to scale their AI efforts while ensuring flexibility and compatibility with various platforms. The API documentation is detailed and user-friendly, making it easier for developers to get started and maintain their custom integrations.
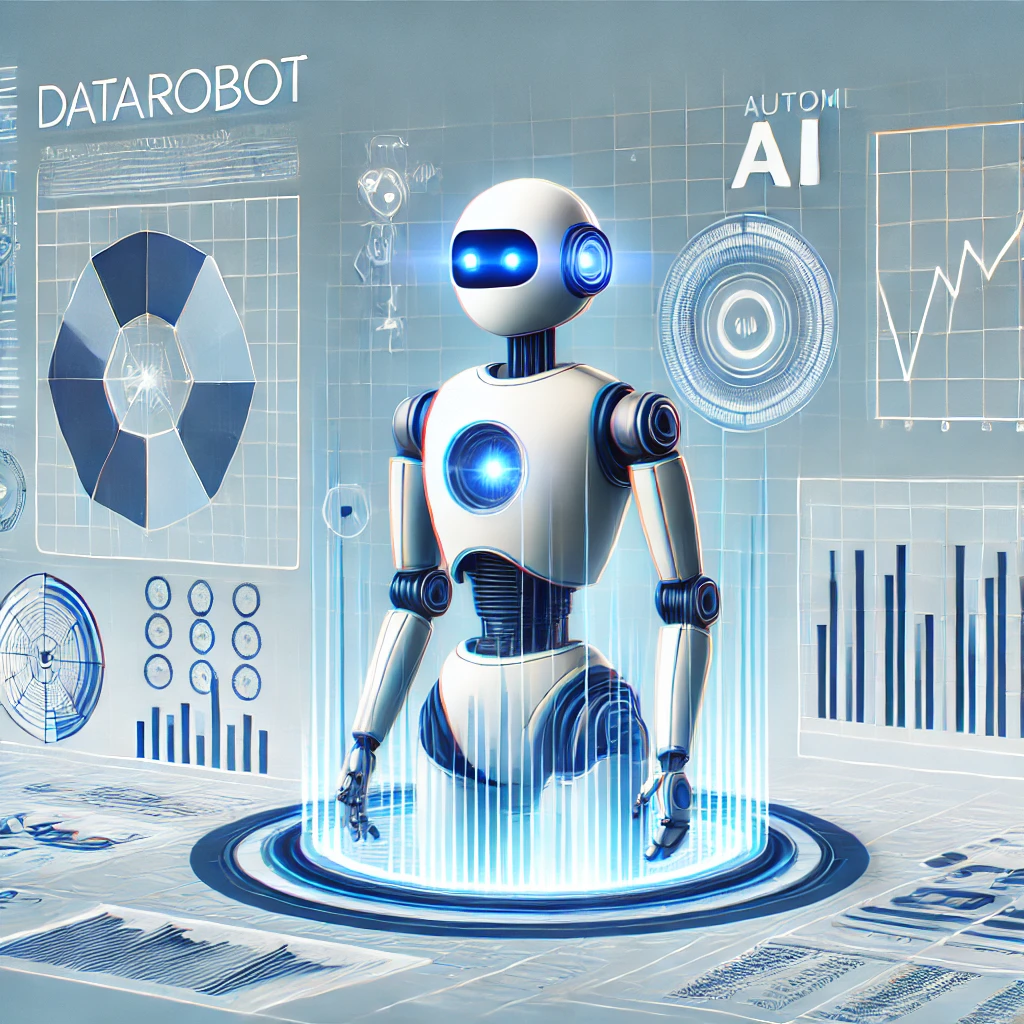
DataRobot in Action: Use Cases
DataRobot has proven to be a game-changer across various industries by providing businesses with powerful AI-driven insights and solutions. In finance, companies use the platform to predict customer behavior, assess credit risk, and detect fraudulent activities, allowing for smarter and faster decision-making. In the healthcare sector, it helps improve patient outcomes by analyzing vast amounts of medical data, identifying patterns, and predicting future health risks, leading to more personalized treatment plans.
Retailers benefit from DataRobot’s ability to forecast demand, optimize pricing, and enhance supply chain management, ensuring they meet customer needs while minimizing costs. Additionally, marketing teams utilize the platform to better understand customer preferences and create targeted, personalized campaigns that improve engagement and increase ROI.
Another significant application is in time series forecasting, where businesses can predict sales trends, resource allocation, and inventory needs, allowing for more efficient operations. The flexibility and automation provided by DataRobot make it a versatile tool across different sectors, from insurance to manufacturing, delivering results quickly and accurately.
Predictive Analytics in Finance and Insurance
Predictive Analytics in Finance and Insurance is transforming how these industries make decisions. By analyzing historical data, predictive models can forecast future trends, identify risks, and uncover opportunities. In finance, predictive analytics helps institutions detect fraudulent activities, optimize investment strategies, and manage credit risk more effectively. For insurance companies, it enhances underwriting processes, improves claims management, and predicts customer churn, enabling personalized pricing and better customer experiences.
DataRobot plays a crucial role in this by automating the creation of predictive models, allowing organizations to generate insights quickly and accurately. It enables finance and insurance companies to leverage machine learning models without needing extensive coding knowledge, speeding up the decision-making process and improving outcomes. With the ability to process vast amounts of data, DataRobot ensures that models are not only accurate but scalable across various use cases.
One of the key benefits of predictive analytics in these sectors is risk management, helping businesses stay ahead in a highly competitive environment.
AI-Driven Customer Insights in Retail and Marketing
Artificial intelligence (AI) is transforming the retail and marketing industries by providing businesses with deeper insights into customer behavior, preferences, and buying patterns. By leveraging machine learning models, companies can analyze vast amounts of data to understand what drives customer engagement and purchase decisions. These insights allow retailers to personalize marketing campaigns, recommend products more effectively, and optimize pricing strategies.
DataRobot enables businesses to easily implement AI-driven solutions that deliver these customer insights without the need for complex coding. Through automated machine learning, retail companies can predict trends, forecast demand, and even anticipate customer churn. Marketing teams can use these insights to create targeted campaigns that resonate more with specific audiences, leading to higher conversion rates and customer satisfaction.
By adopting AI tools like DataRobot, retail and marketing professionals can stay ahead of the competition, adapting quickly to changing market dynamics and consumer behavior.
Machine learning is a key component in turning raw customer data into actionable strategies that drive success.
DataRobot for Healthcare: Improving Diagnostics and Outcomes
In the healthcare industry, artificial intelligence is becoming increasingly important for enhancing patient care, streamlining processes, and improving outcomes. DataRobot helps healthcare professionals leverage AI to analyze vast amounts of data quickly and accurately, leading to better diagnostics and personalized treatment plans. Automating the process of building and deploying machine learning models, enables doctors and medical researchers to focus on interpreting the insights rather than spending time on the technicalities of model development.
One of the key areas where AI can make a significant impact is predictive analytics, allowing healthcare providers to forecast patient outcomes, detect potential risks, and intervene early. For example, machine learning models can predict the likelihood of hospital readmissions or help in diagnosing diseases at earlier stages based on historical patient data. These insights enable healthcare professionals to make more informed decisions, ultimately improving the quality of care and patient experiences.
Additionally, DataRobot integrates well with existing healthcare data systems, ensuring a smooth workflow without the need for extensive technical knowledge. The platform’s ability to handle large datasets ensures that even complex medical data can be processed efficiently. By utilizing AI, healthcare organizations can reduce costs, enhance the accuracy of diagnoses, and improve patient outcomes over time.
Time Series Forecasting for Demand Planning
Time Series Forecasting for Demand Planning is a crucial application in many industries, helping businesses predict future demand for products or services based on historical data. By analyzing patterns over time, time series forecasting models can account for seasonal trends, recurring events, and fluctuations in customer behavior, allowing companies to optimize inventory, staffing, and production.
Using advanced machine learning models, these forecasts become more accurate, improving decision-making and reducing waste. In particular, automated platforms like DataRobot can quickly build and test multiple forecasting models, selecting the one that provides the best predictions based on the available data. This helps businesses react proactively to market changes, minimizing stockouts and overproduction.
One of the key advantages of time series forecasting is its ability to adjust as new data comes in, continuously improving the accuracy of the predictions. With the right tools and models in place, demand planning becomes more precise, ensuring that companies can meet customer demand efficiently.
In today’s fast-paced markets, leveraging forecasting models for demand planning can give businesses a competitive edge by aligning supply with actual demand, reducing costs, and improving customer satisfaction.
Security and Privacy Features
When it comes to Security and Privacy, DataRobot ensures that all data and models are handled with the highest standards. First and foremost, DataRobot uses data encryption both in transit and at rest, ensuring that sensitive information is protected at all times. This encryption is critical for organizations dealing with personal or confidential data.
Additionally, role-based access control (RBAC) allows administrators to define who can access specific resources within the platform. This level of control ensures that only authorized personnel can view or modify datasets, models, and predictions, reducing the risk of unauthorized access.
DataRobot is also compliant with major regulations like GDPR and HIPAA, making it suitable for industries like healthcare and finance, where data privacy is paramount. These compliance measures ensure that the platform follows strict guidelines for handling and processing personal data.
Lastly, audit logging is a key feature that tracks all user actions within the platform. This helps with transparency and provides a clear record for compliance purposes, ensuring that any changes or access to models and data can be traced back to specific users.
Data Encryption and Role-Based Access Control
Data Encryption and Role-Based Access Control are critical features that ensure the security and privacy of data in any AI platform. Data encryption is used to protect sensitive information both at rest and in transit, making sure that data remains inaccessible to unauthorized users. Encryption methods are typically based on advanced algorithms that safeguard the data, ensuring compliance with industry standards and regulations such as GDPR and HIPAA.
Role-Based Access Control (RBAC) is another essential security measure. It allows administrators to define roles and permissions for each user based on their responsibilities within the organization. This means that only authorized individuals can access certain data or perform specific actions, significantly reducing the risk of internal data breaches. By controlling access at a granular level, RBAC ensures that sensitive data and critical processes are only available to those who need them.
Together, these features make the platform both secure and compliant, helping businesses protect their data while maintaining operational efficiency. For organizations handling large volumes of sensitive information, implementing encryption and RBAC is not just a best practice but a necessity.
Compliance with GDPR, HIPAA, and Other Regulations
Compliance with GDPR, HIPAA, and Other Regulations is crucial for any AI and machine learning platform handling sensitive data. DataRobot ensures that users can safely work with data while adhering to important global privacy and security standards. This compliance helps businesses stay protected from legal risks and data breaches.
Here’s a quick overview of the key regulations DataRobot supports:
- GDPR (General Data Protection Regulation)
The GDPR is a European Union regulation that governs data protection and privacy. DataRobot helps companies meet GDPR requirements by providing features like data encryption, access control, and audit logging, ensuring that personal data is handled securely. - HIPAA (Health Insurance Portability and Accountability Act)
HIPAA governs the handling of protected health information (PHI) in the U.S. DataRobot complies with HIPAA, making it suitable for healthcare applications by providing secure environments for managing patient data and maintaining data confidentiality. - SOC 2 (System and Organization Controls)
SOC 2 is a set of criteria that measures a service provider’s ability to securely manage data. DataRobot’s infrastructure is designed to meet SOC 2 standards, ensuring that your data remains safe and secure in cloud environments. - Other Global Privacy Laws
DataRobot also complies with other regional data protection laws like CCPA (California Consumer Privacy Act) and ISO 27001, ensuring broader data security and privacy across multiple regions.
By following these standards, DataRobot provides a secure and compliant environment for businesses that need to manage sensitive data in regulated industries like healthcare, finance, and government.
Ensuring Model Governance and Auditability
Ensuring Model Governance and Auditability is crucial for organizations that want to maintain control over their machine-learning models and ensure they meet regulatory standards. Model governance refers to the processes and frameworks that organizations put in place to manage the lifecycle of AI models, ensuring that these models are transparent, ethical, and compliant with industry regulations.
One key aspect of governance is auditability, which ensures that every action taken with a model—from its creation to deployment and updates—can be tracked and reviewed. This includes monitoring how data is used, how decisions are made by the model, and documenting the changes in the model’s performance over time. Audit trails provide accountability and are especially important in regulated industries like finance and healthcare.
DataRobot helps organizations implement strong governance by offering detailed logging, version control, and role-based access, ensuring that only authorized users can modify or deploy models. Additionally, its explainability tools allow users to understand how models make decisions, which is essential for both internal reviews and external audits.
With proper governance in place, businesses can confidently deploy machine learning models while maintaining compliance with regulations and industry standards.
Continuous Monitoring and MLOps
Continuous Monitoring and MLOps are crucial components for maintaining and improving machine learning models in production. After a model is deployed, its performance can degrade over time due to changes in the underlying data (also known as data drift) or shifts in user behavior. Continuous monitoring ensures that the model’s accuracy, performance, and predictions remain reliable.
MLOps (Machine Learning Operations) refers to the practice of automating and streamlining the deployment, monitoring, and management of machine learning models in production environments. It combines the principles of DevOps with machine learning, allowing teams to track and manage their models more efficiently.
With continuous monitoring, you can set up alerts for anomalies, such as when a model’s predictions start to deviate from expected results. This enables quick action, such as retraining the model with updated data to maintain its performance. MLOps also facilitates automated retraining, version control, and seamless updates to models in production, ensuring that they continue to add value over time.
Platforms like DataRobot make it easy to implement these practices, allowing businesses to manage their models effectively without needing extensive manual oversight. MLOps helps ensure that machine learning models not only function correctly at launch but also remain useful in the long run as new data comes in.
DataRobot’s MLOps: Managing and Monitoring Deployed Models
DataRobot’s MLOps provides a comprehensive solution for managing and monitoring deployed machine learning models in real-time. MLOps, or Machine Learning Operations, ensures that models continue to perform effectively after deployment by enabling continuous tracking, performance evaluation, and retraining when necessary. This system automatically monitors model accuracy, drift in data, and other key metrics, ensuring that predictions remain reliable over time.
One of the key benefits of MLOps is automation. It automates routine tasks such as retraining models when data patterns change or when model performance drops below a certain threshold. This reduces the manual effort typically required to maintain machine learning models in production, allowing businesses to focus more on deriving insights and less on maintenance.
MLOps also ensures transparency and governance by keeping a full audit trail of model changes and updates. This is crucial for industries that require regulatory compliance, such as finance and healthcare, where model governance is essential.
In summary, MLOps in DataRobot allows businesses to seamlessly manage their machine learning models, ensuring they remain accurate and compliant, all while minimizing the resources needed for ongoing model upkeep.
Real-Time Model Monitoring and Alerts
Real-time model monitoring and alerts are crucial features in any machine learning system to ensure models continue to perform accurately over time. Once a model is deployed, its performance can degrade due to changes in data patterns or external factors. Real-time monitoring allows you to track key performance metrics, such as accuracy, precision, and recall, as new data is processed.
When any significant deviation from expected behavior is detected, automated alerts are triggered, allowing data teams to take immediate action. This ensures that models remain reliable and prevents incorrect predictions from affecting business decisions. Additionally, by setting custom thresholds, users can fine-tune when and how alerts are triggered to match the needs of their specific use cases.
With platforms like DataRobot, monitoring is made even easier, as it provides a centralized dashboard to visualize model performance, track drift, and manage alerts. These features help businesses maintain the accuracy of their models without constant manual intervention.
A crucial aspect of this process is model drift, where the model’s performance gradually declines as the underlying data changes.
Continuous Model Improvement and Retraining
Continuous Model Improvement and Retraining refers to the process of regularly updating machine learning models to ensure they stay accurate and relevant as new data becomes available. After deploying a model, its performance may degrade over time due to changes in the underlying data patterns. To address this, retraining the model with new or updated data helps maintain its accuracy and effectiveness.
In platforms like DataRobot, this process is streamlined through automated workflows. The platform can monitor model performance in real-time, triggering retraining when performance drops below a certain threshold. This ensures that models continuously adapt to evolving data without requiring constant manual intervention. Retraining is crucial in industries like finance and healthcare, where data changes frequently and accurate predictions are essential.
By automating model improvement and retraining, businesses can reduce the risk of model drift and ensure their AI systems deliver consistent value.
Getting Started with DataRobot
Getting Started with DataRobot is a straightforward process designed to make machine learning accessible to everyone, regardless of expertise. The first step is to upload your dataset. DataRobot supports various file types, including CSV, Excel, and data from cloud storage services like AWS and Google Cloud. Once your data is loaded, DataRobot automatically cleans and prepares it for analysis, saving you time on data preprocessing.
Next, you select the target variable you want to predict. DataRobot then runs multiple algorithms to find the best-performing model for your data, presenting you with a leaderboard of results. You can review each model’s performance, accuracy, and other key metrics to choose the most suitable one for your needs.
Once you’ve selected a model, deploying it is as easy as clicking a button. You can use the model for real-time predictions or integrate it into your existing systems through APIs. DataRobot also offers detailed documentation and tutorials to guide you through each step, ensuring a smooth start to your machine-learning journey.
Quick Start Guide to Your First AI Model
Getting started with your first AI model using DataRobot is straightforward, even if you’re new to machine learning. Here’s a simple guide to help you build and deploy a model quickly:
- Upload Your Data: Begin by uploading your dataset to the platform. You can use common formats like CSV, and Excel, or directly connect to a data source like a cloud database or data warehouse. DataRobot automatically detects data types, handles missing values, and provides suggestions for cleaning the data.
- Choose Your Target Variable: Once the data is uploaded, select the target variable you want to predict. For example, if you’re working with customer data, your target could be “Churn” (whether a customer will leave or stay).
- Run Automated Model Training: DataRobot’s AutoML feature will take over and automatically select the best algorithms for your data. It evaluates multiple models, ranks them, and provides detailed insights into their performance, including accuracy and speed.
- Evaluate the Results: After the training process, DataRobot provides you with a leaderboard of models. You can evaluate the results, by comparing metrics such as accuracy, precision, and recall. The platform also provides tools for model explainability, helping you understand how your model is making predictions.
- Deploy the Best Model: Once you’re satisfied with the model’s performance, you can deploy it in just a few clicks. DataRobot allows for both real-time and batch predictions, depending on your needs.
- Monitor and Retrain: After deployment, it’s important to monitor your model’s performance to ensure it continues to deliver accurate predictions. If the model’s performance starts to degrade over time, DataRobot can help with automatic retraining to keep it up to date.
With these simple steps, you can build, train, and deploy your first AI model quickly and efficiently. This process empowers you to make data-driven decisions and unlock the potential of AI in your business.
Best Practices for DataRobot Usage
When using DataRobot, following best practices can help you maximize its potential and ensure smooth AI and machine learning workflows.
- Start with Clean, Well-Prepared Data
Data quality is key to successful model performance. Before importing your data into DataRobot, make sure it’s clean—remove missing values, correct inconsistencies, and format the data correctly. Well-organized data leads to better model accuracy and fewer errors during the training process.
- Leverage AutoML Features
DataRobot’s AutoML feature is designed to automate much of the machine-learning workflow. Use this to explore a wide range of models without needing to manually test each one. This saves time and ensures you’re always selecting the best-performing models.
- Use Feature Engineering Tools
DataRobot provides powerful feature engineering capabilities. Take advantage of these to transform your raw data into more meaningful variables that can improve your model’s accuracy. Feature engineering helps to highlight patterns and relationships within your data that might not be obvious at first glance.
- Monitor Models Continuously
Once your model is deployed, regularly monitor its performance. DataRobot offers tools for real-time monitoring to ensure your models continue to perform as expected. This is particularly important when working with changing data environments.
- Optimize Hyperparameters
While AutoML handles much of the heavy lifting, you can fine-tune models further by adjusting hyperparameters. DataRobot allows for hyperparameter optimization to squeeze out extra performance from your models without needing deep technical expertise.
By following these best practices, you can ensure that you’re making the most of DataRobot and its wide range of capabilities to build and deploy effective AI models.
Resources and Tutorials for Learning DataRobot
When learning DataRobot, it’s important to have access to quality resources and tutorials that can guide you through the platform’s features and capabilities. DataRobot University offers a range of free and paid courses designed to help both beginners and experienced users master the platform. These courses cover everything from the basics of machine learning to advanced topics like MLOps, model explainability, and time series forecasting.
For hands-on learners, DataRobot also provides tutorials that walk you through real-world use cases, helping you understand how to apply its tools effectively. The platform’s documentation is another valuable resource, providing detailed instructions on various features and integrations.
Additionally, the community forum is a great place to ask questions, share insights, and learn from other users. It’s an excellent way to get practical advice and keep up with the latest updates and best practices in the world of DataRobot.
DataRobot FAQs: Everything You Need to Know!
DataRobot is an Automated Machine Learning (AutoML) platform that helps businesses build, deploy, and manage AI models quickly and efficiently. It’s popular because it automates the end-to-end AI workflow, making machine learning accessible to both data scientists and non-technical users.
DataRobot automatically tests and ranks multiple machine learning models based on their performance metrics. It evaluates thousands of models using techniques like gradient boosting, deep learning, and decision trees, then suggests the best one for deployment.
Yes! DataRobot is designed for both experts and beginners. Its drag-and-drop interface, automated feature engineering, and built-in explanations make it easy for non-experts to create AI models without coding experience.
Traditional machine learning requires manual data preprocessing, feature selection, model training, and tuning, which can take weeks or months. DataRobot automates these tasks, reducing development time to hours or even minutes while maintaining high accuracy.
DataRobot is widely used in industries such as finance, healthcare, retail, manufacturing, and cybersecurity. For example, banks use it for fraud detection, healthcare providers use it for predicting patient outcomes, and retailers use it for demand forecasting.
DataRobot includes explainable AI (XAI) tools like feature importance, SHAP values, and partial dependence plots to help users understand how models make decisions. This is crucial for regulatory compliance and trust in AI-driven insights.
Yes! DataRobot supports AWS, Azure, and Google Cloud, allowing businesses to train and deploy models in their preferred cloud environment. It also integrates with Databricks, Snowflake, and Hadoop for large-scale data processing.
DataRobot uses optimized computing frameworks and parallel processing to enhance inference speed. It also supports GPU acceleration for deep learning models, ensuring fast predictions even on large datasets.
While DataRobot excels at supervised learning (classification and regression), it also supports unsupervised learning, including clustering, anomaly detection, and time series forecasting.
DataRobot stands out due to its comprehensive automation, enterprise-grade security, model explainability, and ease of use. Unlike many AutoML platforms, it provides end-to-end AI lifecycle management, from data preparation to model monitoring and retraining.
Comprehensive Guide to DataRobot : Audio & PDF
Want to Learn More? Check These Out
Books:
- “Automated Machine Learning: Methods, Systems, Challenges” by Frank Hutter, Lars Kotthoff, and Joaquin Vanschoren
- “Machine Learning Yearning” by Andrew Ng
- “Hands-On Machine Learning with Scikit-Learn, Keras, and TensorFlow” by Aurélien Géron
Websites:
Reviews
There are no reviews yet.